Video Guide
I prepared a detailed guide explaining how to set up and implement this scenario, enabling you to chat with your documents stored in Supabase using n8n.
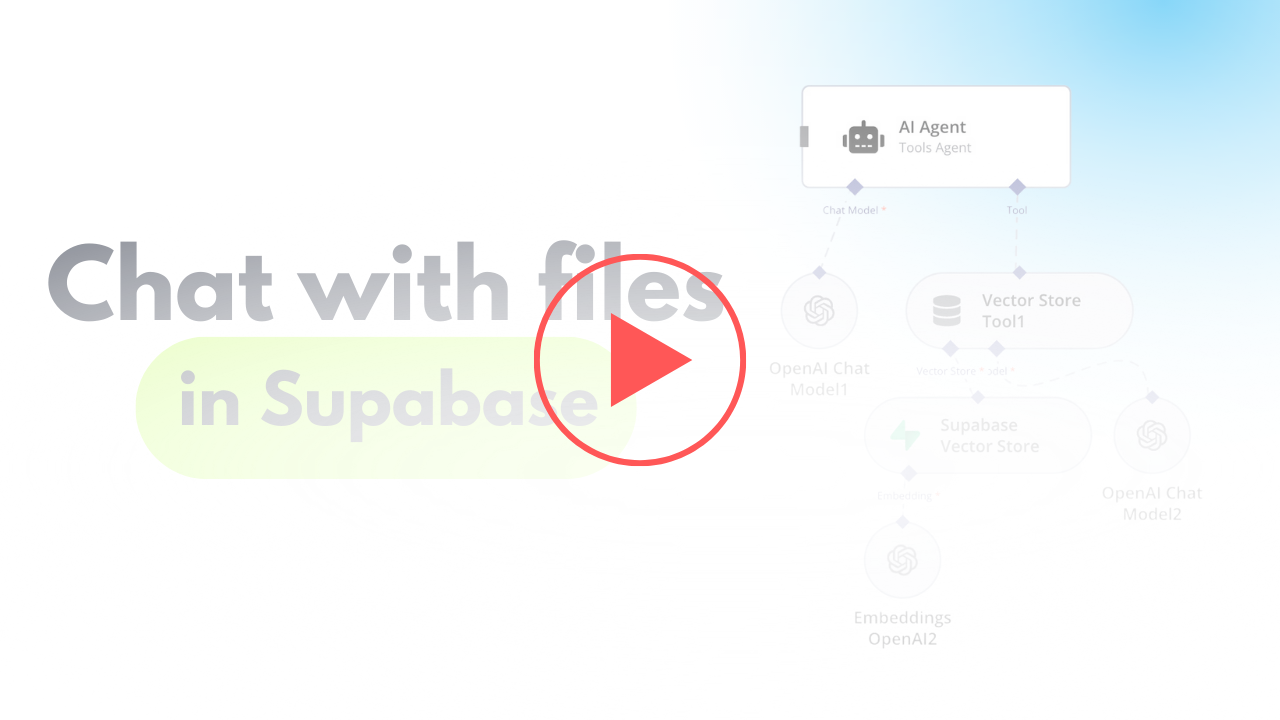
Youtube Link
Who is this for?
This workflow is ideal for researchers, analysts, business owners, or anyone managing a large collection of documents. It's particularly beneficial for those who need quick contextual information retrieval from text-heavy files stored in Supabase, without needing additional services like Google Drive.
What problem does this workflow solve?
Manually retrieving and analyzing specific information from large document repositories is time-consuming and inefficient. This workflow automates the process by vectorizing documents and enabling AI-powered interactions, making it easy to query and retrieve context-based information from uploaded files.
What this workflow does
The workflow integrates Supabase with an AI-powered chatbot to process, store, and query text and PDF files. The steps include:
- Fetching and comparing files to avoid duplicate processing.
- Handling file downloads and extracting content based on the file type.
- Converting documents into vectorized data for contextual information retrieval.
- Storing and querying vectorized data from a Supabase vector store.
- File Extraction and Processing: Automates handling of multiple file formats (e.g., PDFs, text files), and extracts document content.
- Vectorized Embeddings Creation: Generates embeddings for processed data to enable AI-driven interactions.
- Dynamic Data Querying: Allows users to query their document repository conversationally using a chatbot.
Setup
N8N Workflow
-
Fetch File List from Supabase:
- Use Supabase to retrieve the stored file list from a specified bucket.
- Add logic to manage empty folder placeholders returned by Supabase, avoiding incorrect processing.
-
Compare and Filter Files:
- Aggregate the files retrieved from storage and compare them to the existing list in the Supabase
files
table.
- Exclude duplicates and skip placeholder files to ensure only unprocessed files are handled.
-
Handle File Downloads:
- Download new files using detailed storage configurations for public/private access.
- Adjust the storage settings and GET requests to match your Supabase setup.
-
File Type Processing:
- Use a Switch node to target specific file types (e.g., PDFs or text files).
- Employ relevant tools to process the content:
- For PDFs, extract embedded content.
- For text files, directly process the text data.
-
Content Chunking:
- Break large text data into smaller chunks using the Text Splitter node.
- Define chunk size (default: 500 tokens) and overlap to retain necessary context across chunks.
-
Vector Embedding Creation:
- Generate vectorized embeddings for the processed content using OpenAI's embedding tools.
- Ensure metadata, such as file ID, is included for easy data retrieval.
-
Store Vectorized Data:
- Save the vectorized information into a dedicated Supabase vector store.
- Use the default schema and table provided by Supabase for seamless setup.
-
AI Chatbot Integration:
- Add a chatbot node to handle user input and retrieve relevant document chunks.
- Use metadata like file ID for targeted queries, especially when multiple documents are involved.
Testing
- Upload sample files to your Supabase bucket.
- Verify if files are processed and stored successfully in the vector store.
- Ask simple conversational questions about your documents using the chatbot (e.g., "What does Chapter 1 say about the Roman Empire?").
- Test for accuracy and contextual relevance of retrieved results.